This article presents two GPAI-generated definitions for AI models within the meaning of the EU AI Act: A short and a long version. Both are based on the methodological interpretation of the EU AI Act. On the basis of the definition, the sometimes unclear relationship between AI models and AI systems within the meaning of the EU AI Act is examined

- This article contains two definitions for AI models within the meaning of the EU AI Act, created using GPAI tools: a long and a short version. Both variants were checked by hand and slightly post-processed.
- The long version is the result of combining five definitions of AI models created with GPAI tools. Each of the five definitions is based on the legal interpretation of the wording of the EU AI Act.
- At the end of the article, the results are put in relation to the official definition of AI systems within the meaning of the EU AI Act – also with the help of the five GPAI tools.
- At their core, AI systems are “autonomous machines” that interact directly with users. AI models are “computational units” that interact with users only indirectly.
Articles of the EU AI Act mentioned in this post (German)
- Article 3 EU AI Act
Please note that the original text of this article is based on the official German translation of the EU AI Act. Its wording may differ in part from the English version of the EU AI Act.
AI Models and AI Systems under the EU AI Act
The EU AI Act defines the term “AI system” in Article 3 No. 1 EU AI Act. However, there is no overarching definition of “AI models”. And this despite the fact that the term is used differently in many different contexts. In a three-part series of articles, this was addressed and analysed in more detail.
With that in mind, let’s start this article with its end in mind: An overview of what an AI model could be under the EU AI Act and how it relates to AI systems under the EU AI Act.

The question that is answered below: How was this overview created with the help of GPAI tools?
1. Five dimensions of AI models within the meaning of the EU AI Act
The result of the methodological and legal interpretation of the EU AI Act contained in the second part are the following five dimensions of AI models:
- The purpose or usage variance of an AI model.
- The techniques and concepts of AI models.
- The components of an AI model.
- The way AI models are further developed.
- The interfaces of AI models.
The term “dimension” describes criteria that distinguish each AI model in the sense of the EU AI Act in its individual form. It doesn’t matter whether it’s a GPAI model (General Purpose Artificial Intellect), a specific AI model in a high-risk AI, or another AI model. The question of whether open source or closed source is also irrelevant. KI-Modell. Auch die Frage, ob Open Source oder Closed Source vorliegt, spielt keine Rolle.
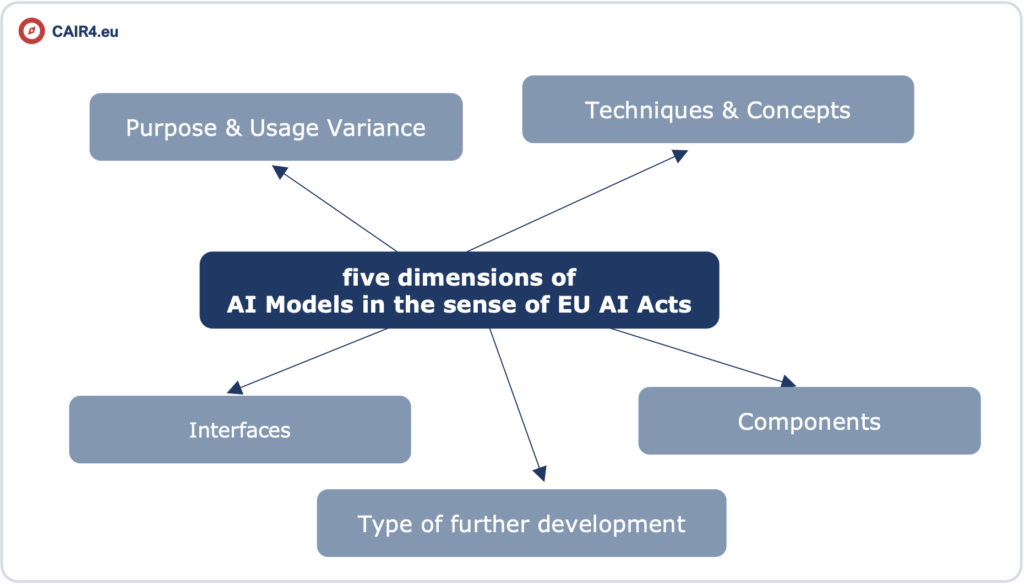

Note the exciting feedback that this article has received from google gemini and Microsoft Copilot. It is shown at the end of this article in the comments!
2. Definitions generated with GPAI
To transform the five dimensions into a textual definition, the help of five leading GPAI tools was enlisted:
- ChatGPT (Browser Application with GPT 3.5)
- ChatGPT (Mac Desktop Application with GPT 4 omni)
- Chat4All (Mac Desktop Application with llama 3.1)
- Microsoft Copilot (Bing Browser Application with GPT 4)
- google gemini (Chrome browser with google gemini AI model family including 1.5 Flash)
An attempt with Mistral German on GPT4All failed. The problems described in the link occurred.

The following note on the Term “GPAI tools”: In particular, Chat4all (= AI system) makes it clear that in the end it is always the combination of AI system plus AI model that creates the results. Therefore, the term “GPAI tools” was used.
2.1 Individual communication with the GPAI tools
The same process was carried out with each of the GPAI tools mentioned:
- First, there was a lengthy conversation of a general nature about AI regulation.
- The respective GPAI tool was then asked to answer the following question:
- “Could you provide a definition of AI models within the meaning of the EU AI Act that integrates the following dimensions?
- purpose or variant of use (specific or GPAI)
- techniques and concepts (AI methodologies)
- components (algorithms, data, parameters)
- development (ability to update, retrain, learn in real time)
- interfaces (interaction with external systems and users)”
The individual answers of the five GPAI tools are listed anonymously at the end of this article under point 5.

With all GPAI tools, restarting a conversation almost always leads to slightly different results for the same question. Sometimes the language or the type of presentation (e.g. number of bullet points) also changes. Therefore, the individual results presented in point 5 are primarily representative answers. They will therefore only be presented at the end of this article.
2.2 Combination of the results
The individual definitions listed under point 5 were compared with each other using ChatGPT 4o and google gemini and summarized in a representative text definition.
- This was done using the following wording: “Create a new definition for AI models within the meaning of the EU AI Act from the five definitions listed above, in which the criteria of all five definitions are summarized.”
- The English result was finally translated into German.
3. The Big Picture: Definition for AI Models
The processing of the five individual definitions has led to the following long version of a definition for AI models within the meaning of the EU AI Act. It was reworked by hand in a few places.
3.1 AI model within the meaning of the EU AI Acts (long version)
The definition below is based on the integration of all five dimensions of AI models that have resulted from the interpretation of the EU AI Act:
An”AI model” is a computational unit designed to process data togenerate targeted predictions, decisions, classifications, or content. AImodels are central building blocks of AI systems.
AI models have five dimensions:
- Purpose or usage variance: AI models are versatile and can be developed for different purposes, from specialized applications such as health diagnostics and financial forecasting to general tasks such as language translation and image recognition. The specific usage determines the design and implementation of the model.
- Techniques and concepts: AI models are based on a variety of techniques and concepts, including machine learning, deep learning, natural language processing (NLP), and computer vision. These methods determine how the model learns, processes data, and makes decisions.
- Components: An AI model consists of several central components: algorithms that form the computational basis; Data that serves as inputs for training and validation; and parameters that are optimized during the training process to maximize performance.
- Evolution: AI models are dynamic systems that can continuously evolve through updates, retraining, and in some cases, real-time learning. This ability to adapt ensures that the model can respond to new data and changing environments.
- Interfaces: AI models interact with external systems via defined interfaces and only indirectly with users. The interfaces enable the integration of the model results into real-world applications, ensure access to predictions and decisions, and enable feedback mechanisms that support the continuous improvement of the model.
3.2 AI model within the meaning of the EU AI Acts (short version)
The following short version of the previous long version was created using the GPAI tools gaoogle gemini, Copilot and ChatGPT 40 in multiple interation and slightly revised by hand:
An “AI model” is a computational unit designed to process data to generate targeted predictions, decisions, classifications, or content. It has one or multi purposes, it uses algorithms, data, and parameters to achieve these results, and it can evolve through updates, retraining, and learning in real time. As a building block of AI systems, AI models only interact indirectly with users.

The short version was specifically designed to be comparable to the official definition of AI models within the meaning of Article 3 No. 1 EU AI Act in terms of the type of wording and the length. This is important because the interaction of this “real” definition with the version recommended for AI models is also important.
3.3 Post-processing
The most difficult challenge of both the long and short versions was the dimension of the interface. Here, there have always been difficulties in differentiating between AI systems within the meaning of the EU AI Acts. These consisted mainly of the frequently encountered statement that AI models interact directly with users. This is not the case. Therefore, this statement was changed manually and provided with the note that AI models only allow indirect communication with users via the AI systems.
4. Interaction of AI model and AI system
The interaction of the definitions for AI models and AI systems is of great importance! This challenge was also addressed using GPAI tools.
First of all, the official definition of AI systems within the meaning of Article 3 No. 1 EU AI Act:
An ‘AI system’ means a machine-based system designed to operate to varying degrees of autonomy and which may be adaptable once it enters service and which derives from the inputs received for explicit or implicit objectives, such as outputs such as predictions, content, recommendations or decisions that may affect physical or virtual environments.
Methodologically, both definitions were entered into ChatGPT 4o. Afterwards, the difference as well as interaction was asked.
4.1 Original answer from ChatGPT 4o
Below is a table created with google gemini and then the original answer from ChatGPT 4o on the interaction of the two definitions:

The difference between the defined AI model definition and the definition of “AI systems” as defined in the EU AI Act lies in the breadth of the scope and the specific aspects that are emphasized:
- Focus and area of application:
- AI model (from definition): An “AI model” is a computational unit designed to process data to generate targeted predictions, decisions, classifications, or content. It has one or more purposes, it uses algorithms, data, and parameters to achieve these results, and it can evolve through updates, retraining, and learning in real time. As a building block of AI systems, AI models only interact indirectly with users.
- AI system (EU AI Act): An AI system, on the other hand, is a more comprehensive machine-based system that is intended to enable autonomous operation to varying degrees. It goes beyond pure model functionality and encompasses the entire system, which can be adaptive once operational to achieve various goals, including the ability to influence physical or virtual environments.
- Autonomy and adaptability:
- AI model: The definition emphasizes the dynamic nature of AI models, especially their ability to evolve through updates, retraining, and real-time learning. This implies a certain adaptability, but the degree of autonomy is not explicitly highlighted.
- AI system: The definition of the EU AI Act particularly emphasizes the degree of autonomy and underlines that the system is adaptable once it is operational. This adaptability is a central feature of the AI system and indicates the ability to make decisions or evolve during operation without human intervention.
- Results and effects:
- AI model: The focus is on how a model makes predictions, decisions, or classifications based on the data entered. It is mentioned that these decisions can influence real-world applications, but the focus is more on the technical functioning and internal processes.
- AI system: The definition of the EU AI Act focuses on the outcomes and the impact of the system’s decisions or predictions on physical or virtual environments. Here, there is more emphasis on the fact that the system’s outputs affect physical or virtual environments, indicating a broader responsibility and impact of the entire system.
To sum up, the definition of the AI model provides a more technical and internal view of the components and functioning of a specific model, while the definition of the AI system in the EU AI Act describes a broader concept that includes not only how it works, but also the autonomy, adaptability, and real-world impact of the system on environments. The AI system is therefore a more comprehensive unit that can include one or more AI models to achieve a goal.
For the three sub-points, the following three tables were also created with google gemini:




As a rule of thumb, an AI system within the meaning of the EU AI Act is an “autonomous machine” that interacts directly with users. The AI model within the meaning of the EU AI Act, on the other hand, is an adaptable “computational unit” that, as part of an AI system, interacts only indirectly with users.
4.2 Final technical evaluation
How should the overall result be assessed? According to the view represented here, the use of GPAI tools to create a definition of AI models within the meaning of the EU AI Act was very helpful: Especially with regard to the different interpretation of the same five dimensions!
On individual aspects of this “cooperation”:
- First of all, it was impressive to see that all the GPAI tools used worked at a similarly high level!
- AI regulation was obviously a well-trained topic for any GPAI tool, which allowed the tools to interpret very precisely in some cases, but also to ask their own questions
- Since all GPAI tools have been given the specification of the five dimensions for AI models, it was also instructive how these were concretized, especially in the long version. As described below under point 5, the definitional approaches are similar in many respects.
- For example, the concept of “computational unit” was brought into play in a similar way by all tools.
- The techniques and concepts were concretized in a very similar way.
- Almost all of them named “algorithms”, “data” and “parameters” as the most important components for AI models.

Nevertheless, a follow-up on the nuances of the definition of AI models was essential. However, in view of the large number of undefined legal terms of the EU AI Act, it can be assumed that GPAI tools can significantly help to evaluate and compare different interpretation results with reasonable effort.
5. The individual GPAI definitions
Abschließend werden die definitorische Langversionen für KI-Modelle aller fünf oben genannten GPAI-Tools dargestellt.

The anonymization was deliberately done in order to focus on the content of the statements and not on the respective GPAI tool. All five tools used worked at a similarly high level.
5.1 First GPAI Definition
An AI model is a computational construct that combines different techniques and
concepts to analyze data, make predictions or decisions, classify patterns,
generate content, or control physical systems. It involves the integration of
multiple components, methodologies, interfaces, and evolutionary capabilities.
- Purpose or use variant:
The main function of an AI model can vary greatly depending on the intended application, such as:- Predictive modeling (e.g. demand forecasting)
- Classification (e.g. spam detection)
- Decision Support Systems
- Content creation (e.g. text-to-image synthesis)
- Control and automation of physical processes
- Techniques and concepts:
AI models use a range of methodologies, including but not limited to:- Machine learning algorithms (supervised, unsupervised, reinforcement learning)
- Deep Learning Techniques
- Neural Networks
- Methods of evolutionary computation
- Symbolic argumentation approaches
- Components:
- Algorithms: Mathematical and computational frameworks that enable the core functions of the AI model.
- Data: Input data used to train or optimize the model, including structured (e.g., tabular), unstructured (e.g., texts, images), or semi-structured formats.
- Parameters: Adjustable settings within the algorithmic framework that affect its performance and behavior.
- Evolution:
- The ability of an AI model to update, retrain, learn in real time allows it to adapt to changing conditions, improve accuracy over time, and integrate new sources of knowledge or data.
- This evolutionary aspect allows the model to refine its predictions, decisions, or control actions based on ongoing feedback.
- Interfaces:
- An AI model interacts with external systems through various interfaces:
- Data entry (e.g. APIs for entering data)
- Output channels (e.g. displays, reports, notifications)
- Control and automation mechanisms
- User-oriented interfaces (e.g. graphical user interfaces, voice assistants)
- An AI model interacts with external systems through various interfaces:
5.2 Second GPAI definition
An “AI model” in the sense of the EU AI Act refers to a unit of computing that has been developed for specific tasks in a defined area and uses data to make predictions. This unit is characterized by:
- Purpose or Variant of Use:
The design and application of the model are determined by the intended area and specific goals, ranging from narrowly focused to more general uses. - Techniques and concepts:
The model uses specific AI methods, such as machine learning and neural networks, that determine its operating principles. - Components:
The model includes various elements, including algorithms, data, and parameters, that work together to make predictions. Data quality and relevance are critical components that influence the performance of the model. - Development:
The model’s ability to evolve—through updates, retraining, or real-time learning—ensures it can adapt to new data or changing needs while meeting regulatory standards.
Interfaces:
The model interacts with external systems and users via defined interfaces and provides predictions and results that can be used within its area of application. These interfaces also include feedback mechanisms that can guide the further development of the model.
5.3 Third GPAI definition
An AI model is a computational system designed to perform tasks that typically require human intelligence. In the context of the EU AI Act, it is a software component that can make predictions or decisions with different degrees of autonomy based on input data and learned patterns.
- Purpose or variant of use:
- AI models can serve a variety of purposes, from image recognition and natural language processing to complex decision-making processes. The EU AI Act categorizes these purposes based on risk levels:
- Minimal risk: Models used in spam filters, video game characters, etc.High risk: Models used in critical infrastructure, law enforcement, education, etc.
- Unacceptable risk: Models that manipulate human behavior, exploit vulnerabilities, etc.
- AI models can serve a variety of purposes, from image recognition and natural language processing to complex decision-making processes. The EU AI Act categorizes these purposes based on risk levels:
- Techniques and concepts
- AI models use various techniques and concepts, including:
- Machine learning: Algorithms that learn from data to make predictions or decisions, without explicit programming.
- Deep learning: A subset of machine learning that uses artificial neural networks with multiple layers.Natural Language Processing (NLP): Enables computer-human interaction in natural language.
- Computer Vision: Allows computers to interpret and understand visual information.
- AI models use various techniques and concepts, including:
- Components:
- Essential components of an AI model include:
- Algorithms: The mathematical procedures that underpin the operation of the model.
- Data: The information used to train and refine the model.
- Parameters: Adjustable values that determine the behavior of the model.
- Essential components of an AI model include:
- Development:
- Updates: Integration of new information or improvements to the model structure.
- Retraining: Modification of model parameters based on new data.
- Real-time learning: Adjust model behavior in response to incoming data without explicit retraining.
- Interfaces:
- AI models interact with:
- External systems: Other software components or hardware devices.
- Users: People who interact directly or indirectly with the model.
- AI models interact with:
5.4 Fourth GPAI definition
An AI model is a computational unit designed to perform specific tasks within a defined range by using data-driven techniques to make predictions, decisions, or classifications. The concept of an AI model under the EU AI Act includes the following essential aspects
- Purpose or variant of use:
- AI models are developed for different purposes, ranging from highly specialized applications to more general functionalities. The intended use of an AI model influences its design, regulatory compliance, and the extent of its use. Depending on the application, AI models may be subject to different risk levels and regulatory scrutiny, especially in high-risk areas such as healthcare, finance, or critical infrastructure.
- Techniques and concepts (AI methodologies):
- AI models use a variety of methodologies, including but not limited to machine learning, deep learning, natural language processing, and computer vision. These techniques form the basic principles that guide the learning process, decision-making skills, and overall behavior of the model. The choice of AI methodologies is closely linked to the purpose of the model and the type of problem it is intended to solve.
- Components (algorithms, data, parameters):
- An AI model consists of several integral components:
- Algorithms: The computational procedures or rules that govern the functioning of the model and allow it to learn from data.
- Data: The input information used to train, validate, and test the model. Data quality, relevance, and diversity are critical to the accuracy and fairness of the model.
- Parameters: The customizable variables within the model that are fine-tuned during the training process to optimize performance.
- Together, these components determine the effectiveness, reliability, and ethical compliance of the model.
- An AI model consists of several integral components:
- Evolution (capacity for updates, retraining, real-time learning):
- AI models are not static; they have the ability to evolve through updates, retraining, or real-time learning. This evolution allows the model to adapt to new data, changing environments, or evolving user needs while ensuring compliance with regulatory standards. Continuous improvement mechanisms are essential to ensure that the model remains effective and relevant over time.
- Interfaces (interaction with external systems and users):
- AI models interact with external systems and end users via defined interfaces. These interfaces facilitate the integration of model outputs into real-world applications, ensuring that predictions, decisions, or classifications are accessible and actionable. Interfaces must also ensure that the AI model operates transparently, securely, and in compliance with data protection regulations. Feedback mechanisms within these interfaces can guide the further refinement and development of the model.
5.5 Fifth GPAI definition
AI models in the context of the EU AI Act
- Purpose or use variant:
AI models are designed to serve a variety of purposes, from generating predictions and recommendations to automating tasks and improving decision-making processes. They can be customized for specific applications such as health diagnostics, financial forecasting, or general tasks such as speech translation and image recognition. - Techniques and concepts (AI methodologies):
AI models use various methodologies, including machine learning (ML), deep learning (DL), natural language processing (NLP), and computer vision. These techniques allow AI systems to learn from data, identify patterns, and make informed decisions. Common concepts include supervised learning, unsupervised learning, reinforcement learning, and neural networks. - Components (algorithms, data, parameters):
AI models consist of several key components:- Algorithms: The mathematical frameworks and procedures that drive the learning and decision-making process.
- Data: The inputs used to train and validate the models, which can include structured data (e.g. databases) and unstructured data (e.g. texts, images).
- Parameters: The customizable elements within the algorithms that are fine-tuned during the training process to optimize performance.
- Evolution (capacity for updates, retraining, real-time learning):
AI models are dynamic and can evolve over time. They have the ability to update and retrain to improve accuracy and adapt to new data. Some models also support real-time learning, which allows them to continuously learn and adapt based on new information and changing environments. - Interfaces (interaction with external systems and users):
AI models interact with external systems and users through various interfaces. These can include APIs (Application Programming Interfaces) for system integration, user interfaces for direct interaction, and communication protocols for exchanging data with other systems. Effective interfaces ensure seamless integration and user-friendly experiences.
Links to the articles of the EU AI Act mentioned in this post (German):
Be First to Comment